AI is changing everything about cybersecurity, for better and for worse. Here's what you need to know
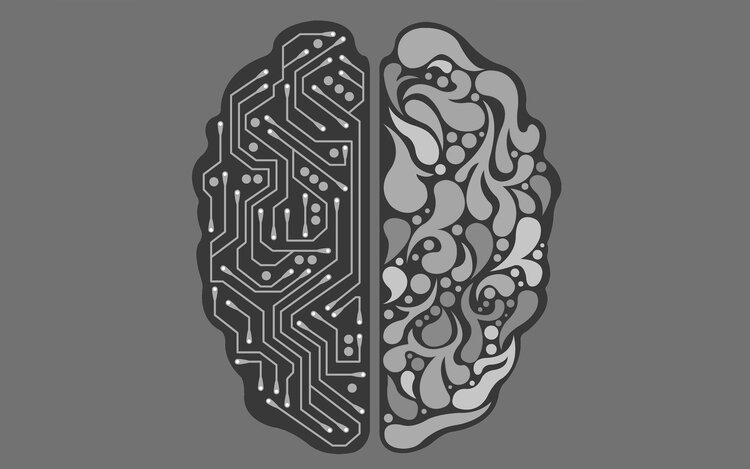.jpg)
2020 has started as 2019 ended, with new cyberattacks, hacking incidents and data breaches coming to light almost every day.
Cyber criminals pose a threat to all manner of organisations and businesses, and the customers and consumers who use them. Some of the numbers involved in the largest data breaches are staggering, with personal data concerning hundreds of thousands of individuals being leaked – each one potentially a new victim of fraud and other cybercrime.
Businesses are doing their best to fight off cyberattacks, but it's hard to predict what new campaigns will emerge and how they'll operate. It's even harder to discern what the next big malware threat will be: the Zeus trojan and Locky ransomware were once major threats, but now it's things like Emotet botnet, the Trickbot trojan and Ryuk ransomware.
It's difficult to defend your perimeter against unknown threats -- and that's something that cyber criminals take advantage of.
AI & ML to the rescue?
Artificial intelligence (AI) and machine learning (ML) are playing an increasing role in cybersecurity, with security tools analysing data from millions of cyber incidents, and using it to identify potential threats -- an employee account acting strangely by clicking on phishing links, for example, or a new variant of malware.
But there is a constant battle between attackers and defenders. Cyber criminals have long tried to tweak their malware code so that security software no longer recognises it as malicious.
Spotting every variation of malware, especially when it is deliberately disguised, is hard: increasingly it's by applying AI and ML that defenders are attempting to stop even the unknown, new types of malware attack.
"Machine learning is a good fit for anti-malware solutions because machine learning is well suited to solve 'fuzzy' problems," says Josh Lemos, vice-president of research and intelligence at Cylance, a BlackBerry-owned, AI-based cybersecurity provider working out of California.
The machine-learning database can draw upon information about any form of malware that's been detected before. So when a new form of malware appears -- either a tweaked variant of existing malware, or a new kind entirely -- the system can check it against the database, examining the code and blocking the attack on the basis that similar events have previously been deemed as malicious.
That's even the case when the malicious code is bundled up with large amounts of benign or useless code in an effort to hide the nefarious intent of the payload, as often happens.
It was these machine-learning techniques that enabled Cylance to uncover -- and protect users against -- a new campaign by OceanLotus, a.k.a. APT32, a hacking group linked to Vietnam.
"As soon as they came out with a new variant in the wild, we knew exactly what it was because we had some machine-learning signatures and models designed to orient to these variants when they appear. We knew they're close enough in their genetic make-up to be from this family of threat," Lemos explains.
But uncovering new kinds of malware isn't the only way machine learning can be deployed to boost cybersecurity: an AI-based network-monitoring tool can also track what users do on a daily basis, building up a picture of their typical behaviour. By analysing this information, the AI can detect anomalies and react accordingly.
"Think about what AI is really good at -- the ability to adapt and respond to a constantly changing world", says Poppy Gustafsson, co-CEO of Darktrace, a British cybersecurity company that uses machine learning to detect threats.
"What AI enables us to do is to respond in an intelligent way, understanding the relevance and consequences of a breach or a change of behaviour, and in real time develop a proportionate response," she adds.
For example, if an employee clicks on a phishing link, the system can work out that this was not normal behaviour and could therefore be potentially malicious activity.
Using machine learning, this can be spotted almost immediately, blocking the potential damage of a malicious intrusion and preventing login credentials being stolen, malware being deployed or otherwise enabling attackers to gain access to the network.
And all of this is done without the day-to-day activity of the business being impacted, as the response is proportionate: if the potential malicious behaviour is on one machine, that doesn't require the whole network being locked down.
Timely response
A key benefit of machine learning in cybersecurity is that it identifies and reacts to suspected problems almost immediately, preventing potential issues from disrupting business.
By deploying AI-based cybersecurity from Darktrace to automate some of the defence functions, the McLaren Formula One team aims to ensure that the network is going to be safe, without relying on humans having to perform the impossible the task of monitoring everything at once.
"If we can't see data coming off the car, if we're compromised, we stop racing -- so high-speed decision-making from machines makes it safer," Karen McElhatton, Group CIO at McLaren explains. "Data isn't just bits and bytes: we have video, we have chats, emails -- it's the variety of that input that's coming and the growing volume of it. It's too much for humans to be able to manage and automated tools are opening our eyes up to what we need to be watching."
That's especially the case when it comes to monitoring how employees operate on the network. Like other large organisations, McLaren employs training to help staff improve cybersecurity, but it's possible that staff will attempt to take shortcuts in an effort to do their job more efficiently -- which could potentially lead to security issues. Machine learning helps to manage this.
"We've got really clever people at McLaren, but with smart people come creative ways of getting around security, so having that intelligence response is really important to us. We can't slow decision-making or innovation down, but we need to enable them to do it safely and securely -- and that's where Darktrace helps us," McElhatton explained.
But while AI and ML do provide benefits for cybersecurity, it's important for organisations to realise that these tools aren't a replacement for human security staff.
It's possible for a machine learning-based security tool to be programmed incorrectly, for example, resulting in unexpected -- or even obvious -- things being missed by the algorithms. If the tool misses a particular kind of cyberattack because it hasn't been coded to take certain parameters into account, that's going to lead to problems.
"Where AI and machine learning can get you into trouble is if you are reliant on it as an oracle of everything," says Merritt Maxim, researcher director for security at analyst firm Forrester .
"If the inputs are bad and it's passing things through it says are okay, but it's actually passing real vulnerabilities through because the model hasn't been properly tuned or adjusted -- that's the worst case because you think you're fully protected because you have AI".
Maxim notes that AI-based cybersecurity has "a lot of benefits", but isn't a complete replacement for human security staff; and like any other software on the network, you can't just install it and forget about it -- it needs to be regularly evaluated.
"You can't assume that AI and machine learning are going to solve all the problems," he says.
Indeed, there's the potential that AI and machine learning could create additional problems, because while the tools help to defend against hackers, it's highly likely that cyber criminals themselves are going to use the same techniques in an effort to make attacks more effective.
AI/ML arms race?
A report by Europol has warned that artificial intelligence is one of the emerging technologies that could make cyberattacks more dangerous and more difficult to spot than ever before. It's even possible that cyber criminals have already started using these techniques to help conduct hacking campaigns and malware attacks.
"A lot of it is, at the moment, theoretical, but that's not to say that it hasn't happened. It's quite likely that it's been used, but we just haven't seen it or haven't recognised it," says Philipp Amann, head of strategy for Europol's European Cybercrime Centre (EC3).
It's possible that by using machine learning, cyber criminals could develop self-learning automated malware, ransomware, social engineering or phishing attacks. Currently, they might not access to the deep wells of technology that cybersecurity companies have, but there's code around that can provide cyber criminals with access to these resources.
"The tools are out there -- some of them are open source. They're freely available to everyone and the quality is increasing, so it's likely to assume that this will be part of a criminal's repertoire if it isn't already," Amann says.
While it may be unclear if hackers have used machine learning to help develop or distribute malware, there is evidence of AI-based tools being used to conduct cybercrime; last year it was reported that criminals used AI generated audio to impersonate a CEO's voice and trick employees into transferring over €220,000 ($243,000) to them.
This 'deepfake voice attack' added a new layer to business email compromise scams, in which attackers claim to be the boss and request an urgent transfer of funds. This time, however, the attackers used AI to mimic the voice of the CEO and request the transfer of funds.
The nature of a CEO's job means their voice is often in the public domain, so criminals can find and exploit voice recordings and -- and this case isn't the only example.
"Other industry partners have confirmed other cases that haven't been reported; so that's where we know an AI-based system has been used as part of a social-engineering attack," says Amann.
AI-based deepfake technology has already caused concern when it comes to spreading disinformation or even abuse of individuals via fake videos, leading to calls for deepfake regulation.
But the problem here is that while most people would see this as a warning not to meddle in this field, cyber criminals will simply look to take advantage of any technology in order to make money from malicious hacking. For example, machine learning could be employed to send out phishing emails automatically and learn what sort of language works in the campaigns, what generates clicks and how attacks against different targets should be crafted.
Like any machine-learning algorithm, success would come from learning over time, meaning that it's possible that phishing attacks could be driven in the same way security vendors attempt to defend against them.
"There's a whole cyber criminal element here for financial gain, which can leverage AI and machine learning effectively," warns Lemos.
However, if AI-based cybersecurity tools continue to develop and improve, and are applied correctly alongside human security teams, rather than instead of them, this could help businesses stay secure against increasingly smart and potent cyberattacks.
"One thing we can be certain of is the offices of tomorrow aren't going to like those of past. AI is how technology responds to our ever-changing world. It updates automatically and learns how humans react. We can't second-guess technology, but we can watch it and learn from it and adapt," says Gustafsson.
"You could move into a world where your whole cybersecurity posture is enhanced, with the ultimate vision being you could end up with a self-learning and self-healing network that can learn negative behaviours and stop them happening," she says.
source zdnet
Industry: Cyber Security